High-Fidelity GAN Inversion for Image Attribute Editing
-
Tengfei Wang
HKUST
  -
Yong Zhang
Tencent AI Lab
  -
Yanbo Fan
Tencent AI Lab
  -
Jue Wang
Tencent AI Lab
  -
Qifeng Chen
HKUST
 
Abstract
We present a novel high-fidelity generative adversarial network (GAN) inversion framework that enables attribute editing with image-specific details well-preserved (e.g., background, appearance, and illumination). We first analyze the challenges of high-fidelity GAN inversion from the perspective of lossy data compression. With a low bit-rate latent code, previous works have difficulties in preserving high-fidelity details in reconstructed and edited images. Increasing the size of a latent code can improve the accuracy of GAN inversion but at the cost of inferior editability. To improve image fidelity without compromising editability, we propose a distortion consultation approach that employs a distortion map as a reference for high-fidelity reconstruction. In the distortion consultation inversion (DCI), the distortion map is first projected to a high-rate latent map, which then complements the basic low-rate latent code with more details via consultation fusion. To achieve high-fidelity editing, we propose an adaptive distortion alignment (ADA) module with a self-supervised training scheme, which bridges the gap between the edited and inversion images. Extensive experiments in the face and car domains show a clear improvement in both inversion and editing quality.
Results on High-Fidelity Image Editing
Original image (left) and edited image (right).
![]() |
![]() |
![]() |
![]() |
![]() |
![]() |
![]() |
![]() |
![]() |
![]() |
![]() |
![]() |
![]() |
![]() |
![]() |
![]() |
![]() |
![]() |
![]() |
![]() |
![]() |
![]() |
![]() |
![]() |
![]() |
![]() |
![]() |
![]() |
![]() |
![]() |
![]() |
![]() |
Results on High-Fidelity Video Editing (+ Smile)
Approach
Overview of our high-fidelity image inversion and editing framework. The basic encoder E0 infers a low-rate latent code W corresponding to a low-fidelity reconstruction image hat{X}o. The distortion map contains the lost high-frequency image-specific details to improve the reconstruction fidelity. The red dotted boxes indicate the editing behaviour with certain semantic direction. To achieve high-fidelity image editing, we propose the distortion consultation branch to facilitate the generation. In the distortion consultation, Δ is first aligned with the low-fidelity edited image by ADA and then embedded to a high-rate latent map C via the consultation encoder Ec. Latent code W and latent map C are combined via the consultation fusion (see details in the right part) across layers of G0 to generate the final edited image.
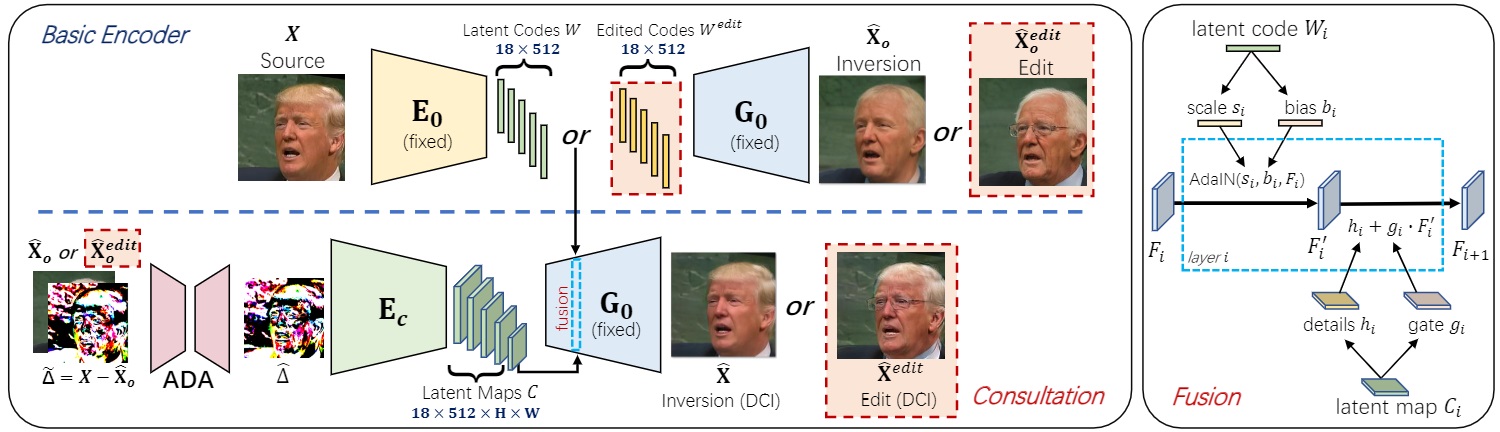
More Results
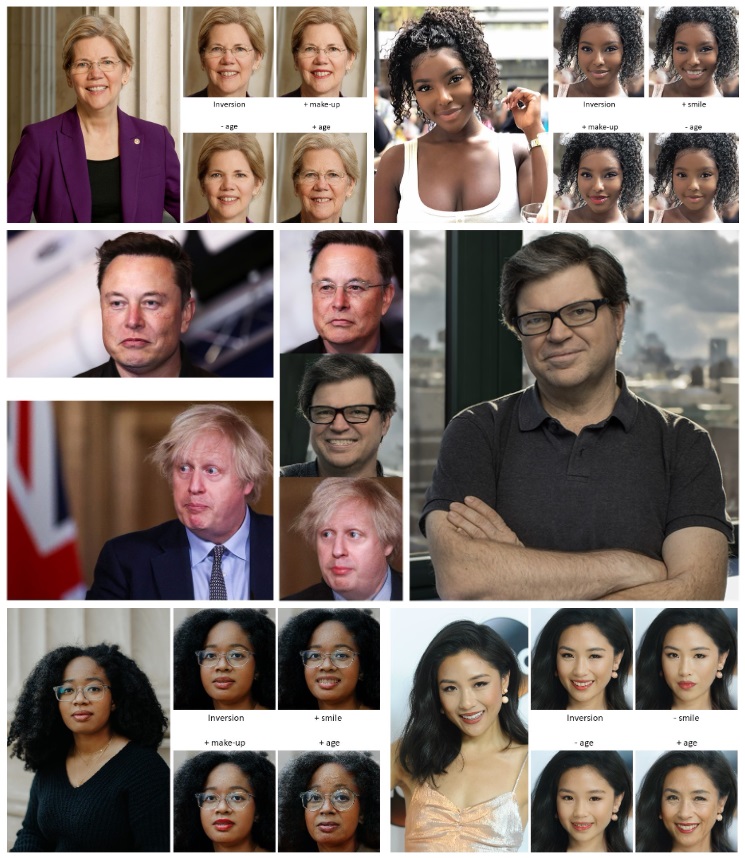
BibTeX
@inproceedings{wang2021HFGI, title={High-Fidelity GAN Inversion for Image Attribute Editing}, author={Wang, Tengfei and Zhang, Yong and Fan, Yanbo and Wang, Jue and Chen, Qifeng}, booktitle = {Proceedings of the IEEE/CVF Conference on Computer Vision and Pattern Recognition (CVPR)}, year={2022} }